Randomization in social and clinical experiments is generally accepted as the “gold standard” for causal conclusions, because it balances baseline covariates across treatment groups on average, yielding unbiased causal effects. However, although randomization balances baseline covariates on average, it is possible that covariates could still be imbalanced just by random chance, compromising the validity of results. Although chance imbalance is often thought of as a rare, unlucky occurrence, it actually is quite common. For example, with only 10 independent covariates, there is a 40 percent chance that at least one will be significantly (using α = 0.05) different at baseline, just by random chance! Why subject your RCT to this kind of risk? If baseline covariates are thought to matter, balance should be checked at the time of randomization, before the experiment is conducted, and allocations yielding unacceptable balance should be eliminated.
Rerandomization (Morgan and Rubin, 2012) provides a way to avoid this chance imbalance for baseline covariates available at the time of randomization. Rerandomization works by checking balance at the time of randomization and rerandomizing if balance is unacceptable according to pre-specified criteria for acceptable balance. This process continues until an allocation with acceptable balance is achieved, and only then is treatment actually administered. When the criteria for acceptable balance is objective and specified in advance, and when treatment groups are equally sized, rerandomization maintains overall unbiasedness while also guarding against conditional bias due to chance imbalance. Thus we preserve the “gold standard” benefits of randomization, while avoiding detrimental chance imbalances; an idea Tukey (1993) called the “platinum standard.”

Although the original motivation was to guard against confounding, by improving covariate balance, rerandomization also improves precision when outcomes are correlated with the covariates being balanced. However, to take advantage of these gains in precision, analysis must reflect the rerandomization procedure, for example by randomization-based inference. Not accounting for rerandomization in analysis will still result in “valid” results in the sense that significant p-values can be trusted, the Type I error rate will no larger than as stated, and confidence intervals will have at least the nominal coverage. However, results will be conservative, meaning that p-values could be smaller and intervals could be narrower if the rerandomization were taken into account.
We are currently working on the evaluation of an educational intervention that used rerandomization to assign teachers across five large, urban school districts to treatment or control. Although data collection took place in 2016-17, the treatment started with a four-day “Summer Institute” and so treatment assignment had to take place before we had the 2016-2017 students’ baseline data; the enrolled teachers’ 2015-2016 students’ baseline data was the only alternative. The rerandomization criteria enforced balance on two covariates: a composite measure of standardized test scores and a composite measure of socio-economic status. We conducted randomization independently within each district, and the rerandomization criteria—both the variables used for the composite measures and the exact criteria for acceptable balance—differed slightly from district to district.
In Figure 2, we show, for one of the five districts, the resulting improvement in balance using rerandomization rather than pure randomization (ignoring the covariates). Zero represents perfect mean balance (equal means in treatment and control groups), and the rerandomization yields a distribution with covariate difference in means more closely concentrated around zero, with no extreme differences. The balance for the actual experiment is depicted with a black dot, and is very good for both covariates, as enforced by rerandomization.
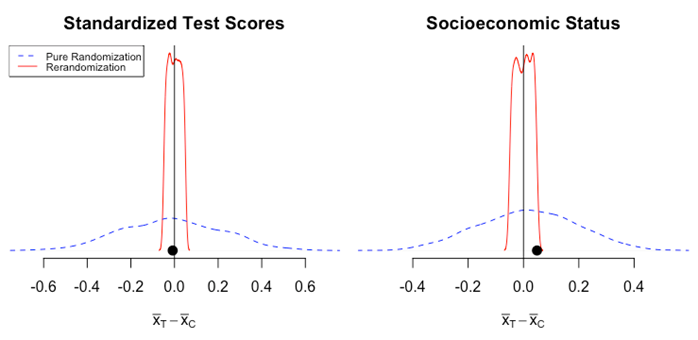
Although we do not have outcome data yet, the amount to which rerandomization will decrease the variance of the outcome difference in means (tightening the distribution around the truth) depends both on the amount of improvement in covariate balance (as shown in Figure 2) and on the extent to which the outcome is correlated with the covariates, as measured by R2. Given this level of covariate balance, the resulting precision of the outcome difference in means would increase by a factor of 2 if R2 = 0.53 or 3 if R2 = 0.68, equating to roughly doubling or tripling the sample size! This example illustrates the benefits of rerandomization for an educational intervention, but rerandomization can improve baseline balance and outcome precision for any field or study utilizing randomization.
You must be logged in to post a comment.